A fraud rules engine is an analytical or systematic approach employed by financial institutions to detect and prevent fraudulent activities by applying predefined rules and criteria. The concept behind rules engines is straightforward: transactions are analysed by a system that identifies specific patterns. These rules are based on historical data, patterns (modus operandi, as it is called in the financial crime world), and specific triggers that flag potentially suspicious activities.
For instance, a rule might specify that any transaction exceeding $500 should undergo manual review, or that logins from high-risk geographic locations should be scrutinized. These rules can consider a wide range of factors, including the type and price of the item, time zone, geographic location, address details, and phone information. Transactions can then be automatically approved, rejected, or flagged for manual review based on these rules.
While advancements in machine learning and artificial intelligence have revolutionized fraud detection, the rules engine model continues to be a crucial component of many banks’ fraud prevention frameworks.
How a Fraud Rules Engine Works
A fraud rules engine operates by applying a series of rules to each transaction processed by the bank. These rules are based on:
- Thresholds: Transactions exceeding certain monetary limits are flagged. For instance, a transfer above $10,000 might trigger an alert for further scrutiny.
- Patterns: Unusual transaction patterns, such as multiple transactions in a short period, can indicate potential fraud. For example, if a customer makes several large withdrawals within a few minutes, this could be suspicious.
- Behavior: Changes in customer behaviour, like a sudden increase in spending or transactions in high-risk locations, can trigger alerts. For instance, if a normally low-spending customer suddenly makes a large purchase in a foreign country, this could be flagged.
- Anomalies: Deviations from typical transaction behaviours, such as unusual login times or locations, are monitored. For example, if a customer’s account is accessed from a different country within a short period of time, this might be considered suspicious.
When a transaction meets one or more of these criteria, it is flagged for further investigation. The bank’s fraud analysts then review the flagged transactions to determine if they are indeed fraudulent.
Components of a Fraud Rules Engine
1. Rule Definitions:
- Rules are the core of the fraud rules engine. They are defined based on historical fraud data, regulatory requirements, and known fraud patterns. For example, a rule might state that any transaction over $5,000 made from a high-risk country should be flagged.
2. Data Integration:
- The engine integrates data from multiple sources, including transaction histories, customer profiles, and external databases. This comprehensive data integration enhances the accuracy of the engine.
3. Real-Time Processing:
- Modern fraud rules engines process transactions in real-time. This immediate analysis allows banks to quickly detect and respond to suspicious activities.
4. Alert Management:
- When a rule is triggered, the system generates an alert. These alerts are prioritized based on the level of risk and sent to fraud analysts for review.
5. Case Management:
- The engine includes a case management system to track the investigation of flagged transactions. Analysts can document their findings and actions taken, ensuring a thorough and traceable investigation process.
6. Reporting and Analytics:
- The system generates reports on the performance of the rules, including metrics such as false positives, detection rates, and overall fraud prevention effectiveness. This data is used to refine and update the rules continually.
7. Compliance Monitoring:
- Ensuring adherence to regulatory requirements is a critical function. The engine tracks and reports on compliance-related activities, helping banks meet their legal obligations.
Rules Engines Are Still Relevant
Despite the advancements in AI and ML, rules engines continue to be widely used in fraud detection for several reasons:
- Simplicity and Transparency: Rules engines are straightforward to understand and implement. The rules are clear and can be easily explained to regulators and stakeholders.
- Regulatory Compliance: Many regulations require specific criteria to be monitored. Rules engines can be tailored to meet these regulatory requirements effectively.
- Complementary to AI: Rules engines can work alongside AI systems, providing a multi-layered approach to fraud detection. While AI can identify complex patterns, rules engines ensure that straightforward, known risks are consistently monitored.
Types of Fraud systems
There are a several types of fraud-based systems, including AI or machine learning systems; however in reality the ones which are most commonly used today in Government, large banks and institutions are
Risk-Based vs. Rules-Based Fraud Systems
Risk-Based Fraud Systems:
- Dynamic Approach: Risk-based systems assess the risk of each transaction dynamically based on various factors, such as transaction history, customer profile, and current context.
- Adaptive: These systems can adapt to new fraud patterns and learn from each transaction, improving their effectiveness over time.
- Scoring: Each transaction is given a risk score, and actions are taken based on the level of risk. High-risk transactions may be flagged for review, while low-risk ones are processed normally.
Rules-Based Fraud Systems:
- Static Approach: Rules-based systems apply predefined rules to each transaction. These rules do not change dynamically and are based on historical data and known fraud patterns.
- Predictable: The outcomes are predictable, and the rules are clear and easy to understand.
- Compliance-focused: These systems are excellent for meeting specific regulatory requirements and ensuring consistent monitoring.
Comparison:
Feature | Risk-Based System | Rules-Based System |
Approach | Dynamic and adaptive | Static and predefined |
Learning Capability | Continuously learns from new data | Fixed rules, requires manual updates |
Flexibility | High, adjusts to new fraud patterns | Low, needs regular rule updates |
Predictability | Less predictable, depends on dynamic scoring | Highly predictable and transparent |
Regulatory Compliance | Good for adaptive risk management | Excellent for specific compliance criteria |
Building an Effective Fraud Rules Engine
1. Define Objectives:
- Clearly outline the goals of the fraud rules engine, such as reducing fraud losses, complying with regulations, and protecting customer assets.
2. Develop Rules:
- Create rules based on historical data, known fraud patterns, and regulatory requirements. Ensure that these rules are specific, actionable, and relevant.
3. Implement Technology:
- Use a robust technology platform that supports the implementation and management of fraud rules. Ensure the platform can handle real-time analysis and alerting.
4. Integrate Data Sources:
- Ensure the fraud rules engine can access and analyse data from various sources, including transaction histories, customer profiles, and external databases.
5. Monitor and Update:
- Continuously monitor the performance of the fraud rules engine and update the rules as needed to adapt to new threats and changing regulations.
6. Train Employees:
- Provide training for fraud analysts and other relevant staff on how to use the fraud rules engine effectively and how to interpret the alerts generated.
By implementing such specific rules, banks can enhance their fraud detection capabilities and protect both their assets and their customers from fraudulent activities.
A few use cases and examples of these rules are:
1. Multiple Transactions in a Short Timeframe:
- Rule: If a customer makes more than three transactions within a ten-minute period, the transactions should undergo manual review.
2. Unusual Spending Pattern:
- Rule: If a customer’s spending in a single day exceeds their average daily spending by 200%, flag the transaction for review.
3. High-Risk Merchant Category Codes (MCC):
- Rule: Transactions involving merchant category codes associated with high-risk businesses, such as gambling or adult entertainment or certain high risk crypto currency firms, should be flagged for review. We do this as certain business categories are more prone to fraud than others. This rule ensures heightened scrutiny of transactions related to these categories.
4. Cross-Border Transactions:
- Rule: If a transaction is initiated from a country different from the customer’s usual country of residence, it should undergo additional verification. Though this might be a genuine case of the customer travelling cross-border, it can also be a red flag for fraud, especially if the transactions are unexpected and inconsistent with the customer’s usual activity.
5. Inconsistent Device Information:
- Rule: If a customer attempts to log in using a device or IP address that has not been previously associated with their account, the transaction should be given a higher score and if it is coming from a device which has seen fraud previously, then it should be flagged for further review and most probably the transaction might be rejected.
6. Mismatch in Shipping and Billing Addresses:
- Rule: Transactions where
A) the shipping address significantly differs from the billing address or
B) the distance between them is a lot
So, they are given a higher score by the fraud system. This rule especially helps when fraudsters often use stolen Credit card information to receive goods.
7. ATM Withdrawals in Quick Succession:
- Rule: If a customer makes more than two ATM withdrawals within a 30-minute period, the account should be flagged for review, as rapid ATM withdrawals can indicate card skimming or other types of fraud. Monitoring these transactions helps mitigate risks.
8. Large Transaction Without Previous History:
- Rule: If a customer who usually makes small purchases suddenly makes a large transaction (e.g., above $1,000), flag it for review.
That’s Rules Engines and there are a few different types of them and scenarios for how they are used.
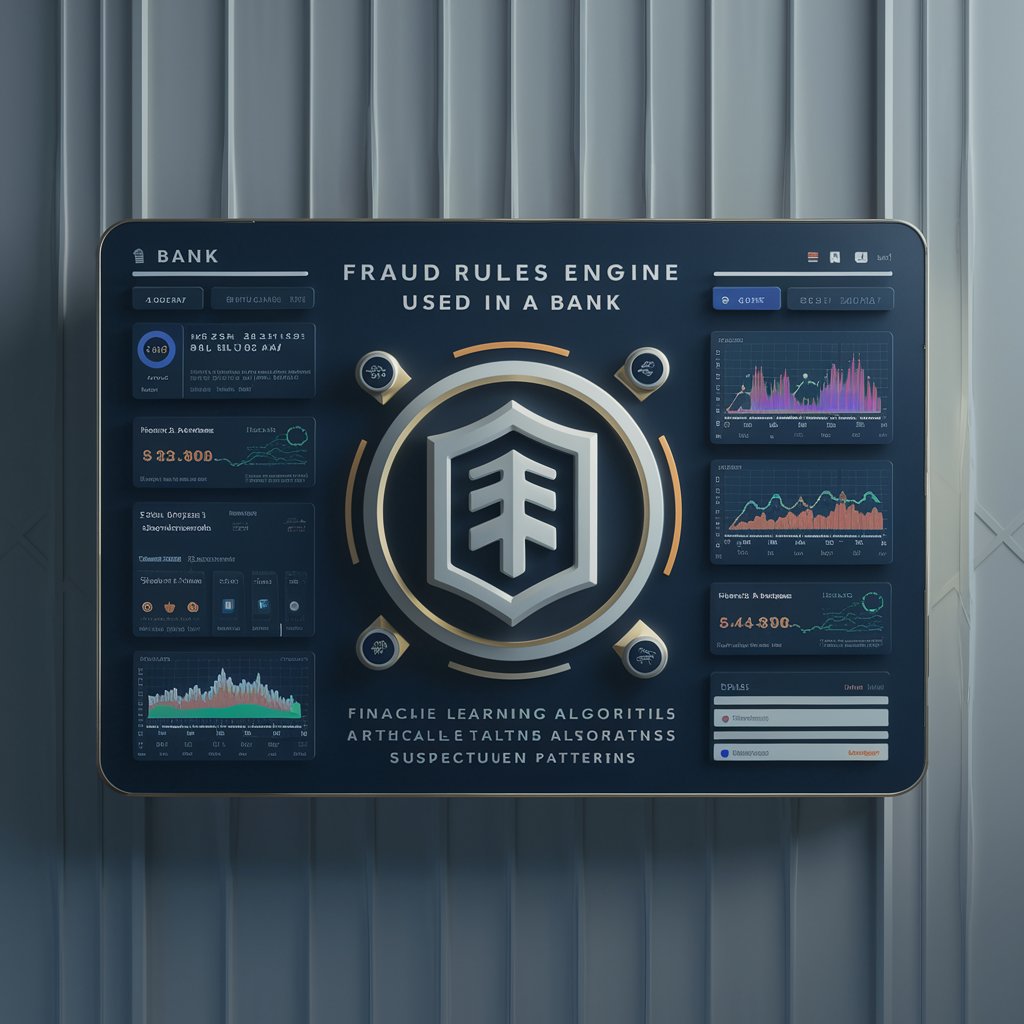